A dataset to advance wildlife monitoring with drones
With “Bucktales” three postdocs hope to power a wave of collaboration on AI tools for drone-based study of wild animals

Biologists are increasingly deploying cameras on drones to study animals in nature. The remote-controlled vehicles are an easy and cheap way to record activity of animals with minimal disturbance. But as drone usage has grown in the biological community, so too have the problems associated with studying the video data.
Video data is big data. A biologist flying a drone above the Serengeti must sift through hours of footage before she can answer a fundamental question such as, “How many rhinos are there?” Machine learning algorithms have become crucial for automating routine tasks, such as identifying animals or tracking animal movement, so that video can be analyzed efficiently. But developing such algorithms is a labor that few biologists have the training—or heart—to do.
Computer scientists, in contrast, relish the challenge. Some have developed animal tracking algorithms using publicly available videos from platforms like YouTube. But these offerings have yet to deliver solutions to real-world biological problems. “Animal videos scraped from the internet just can’t reproduce the complex visual problems we find in nature,” says Hemal Naik, a postdoc in the Department for the Ecology of Animal Societies (EAS) at MPI-AB.
Naik is part of team MELA, which includes postdocs Vivek Hari Sridhar and Akanksha Rathore. Team MELA work in sanctuaries in India to study blackbuck, a species of antelope, which gather in huge groups during the mating season. To learn more about this rare behavior, the team fly a fleet of three drones that record interactions among the entire blackbuck aggregation from 80 meters in the air.

“I’ve got video of two hundred antelopes running around like crazy,” says Naik. “I need an algorithm that can distinguish very similar looking individuals and keep track of their movements. But which of the off-the-shelf algorithms out there can give me the accuracy that I want?”
There was no answer to this question, so team MELA created a solution to find out. Their dataset, presented on December 10 at the NeurIPS conference, is the first that allows biologists to test the accuracy of algorithms that identify and track animals in drone footage.
A different dataset
“You can think of this dataset as a race track,” adds Naik. “If there is an algorithm that you want to use to identify and track animals in drone video, come and test it with our dataset to see how accurate it is.”
The “Bucktales” database is drawn from more than 60 hours of drone footage taken of blackbucks in a sanctuary in Rajasthan, India. It is the only published dataset of wild animals recorded using multiple drones. Of those 60 hours, the team manually annotated approximately 12 minutes of footages with special focus on long sequences. These annotations mean that the identity and trajectories of hundreds of individual blackbucks have been confirmed by human observation or “ground truthing,” which is the essential step to train algorithms that can automate the process of identifying and tracking animals.
“This was the back breaking part of the work,” says Akanksha Rathore. “In algorithmic development, twelve minutes of annotated video is very, very long. It involved a team of domain experts annotating over a million bounding boxes to create this dataset. One of the co-authors also created a ‘How-To’ video so other biologists can follow these steps to create their own dataset,” she says.
The effort allowed the team to “benchmark” seven of the current state-of-the-art algorithms available for identifying and tracking animals in video. “We show which algorithm works with the best accuracy for now, and also highlight challenges that need to be solved to improve the algorithms,” says Naik. A challenge that the study identified is that of processing time—current software options require eight hours to process just three minutes of video.
With their unique annotated and benchmarked dataset, the authors hope they can open the door for computer scientists to step in to help solve these challenges.
Crowdsourcing computer scientists
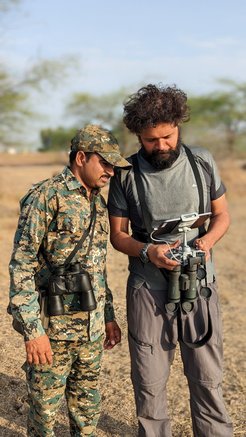
“It’s biologists crowd sourcing algorithmic development from computer scientists,” says Sridhar. “Video-based animal tracking and identification faces hard problems that biologists can’t solve on our own. We hope that by doing the hard work of annotating and benchmarking a very large dataset of animal videos, we can invite computer scientists to contribute positively to some of the biggest biological problems of our time.”
The Bucktales dataset, focused only on antelope, is the first step in what the authors hope will be an ecosystem of annotated databases. “We want to build a much larger system that works generically across taxa,” says Naik. “Then biologists can pick algorithms off the shelf because the methods will be tested on different animals.”
This algorithmic diversity can pave the way for drone-based video to be part of protecting the planet’s biodiversity. The ultimate goal would be for wildlife managers to be able to monitor threatened species by flying out a fleet of cheap, easy-to-use drones.
In India’s blackbuck sanctuaries, rangers are eagerly awaiting the day when this is possible. “The park authorities keep asking us if an algorithm exists that can automatically count blackbuck in videos,” says Sridhar. “They need to conduct annual census of the protected blackbuck population, but they have few resources to do so.”
Automated analysis of drone footage would solve the problem.
“We’re still far off that dream, but our published dataset is the first step to make it a reality,” he says.