Revealing the structure of sensory interaction networks in animal groups
We still have only a relatively rudimentary understanding of how individual cognition relates to collective decision-making, and more generally to the ‘computational properties’ of groups. Collective properties often result from dynamic processes that occur across multiple scales of organization. For complex biological systems, including animal groups, it is seldom possible to observe directly the underlying interaction network architecture. Consequently, our understanding of how individuals, and collectives, encode and process information is based on untested, and currently untestable, assumptions. Conventional models of collective animal behavior typically regard individuals as “self-propelled particles”, and these fail to account for many important information-processing capabilities of real groups, such selective amplification of response.
In order to get closer to the actual sensing capabilities of organisms, and to ask how information is integrated at multiple scales, we have been developing software that allows us to track not just fishes’ positions, but that can also reconstruct (automatically) their body posture, and their visual field, allowing us to ‘see’ the world from the perspective of the organisms themselves. An example of this is shown below for fish (golden shiners) that swim in a relatively ‘planar’ 2D school (we are currently working on implementing this in 3D).
Vision is known to be especially important for many diurnal freshwater species grouping since it allows fast, and relatively long range, social interactions. For our golden shiners, reducing light levels completely inhibits schooling, whereas ablation of the ‘lateral line’ that senses hydrodynamic cues appears to play a very minor role.
We have demonstrated that visual sensing networks are much better descriptors of how information (encoded via behavioral change) flows, as waves, through these groups under two ecologically-relevant scenarios:
1. Leadership during food-finding
Strandburg-Peshkin, A., Twomey, C.R., Bode, N.W., Kao, A.B., Katz, Y., Ioannou, C.C., Rosenthal, S.B., Torney, C.J., Wu, H., Levin, S.A. & Couzin, I.D. (2013) Visual sensory networks and effective information transfer in animal groups, Current Biology 23(17), R709-711.
2. Collective evasion response
Rosenthal, S.B., Twomey, C.R., Hartnett, A.T., Wu, H.S. & Couzin, I.D. (2015) Revealing the hidden networks of interaction in mobile animal groups allows prediction of complex behavioral contagion, PNAS 112(15), 4690-4695.
Explicitly taking into account the visual nature of interactions (i.e. who can see whom within the group, and how much) provides a better predictor of information transfer than do more traditionally-used interaction models, such as those that assume individuals interact with all neighbors within a fixed distance (metric interactions) or with a fixed number of nearest neighbors (topological interactions). Moreover, we show that these visual interaction networks are structurally different from those specified by metric or topological assumptions, with potentially important consequences for information transfer.
We have also been able to establish how the complex social scene is employed by individuals (i.e. which visual features they employ when making movement decisions) which allows us to reveal the “hidden” complex communication network via which behavioral change spreads rapidly through groups. This has allowed us to much better understand the relationship between the structure of interaction networks and the spread of social contagion (and demonstrated key differences with previous theoretical predictions) allowing us to predict the magnitude of behavioural cascades before they even occur.
Ongoing and future work
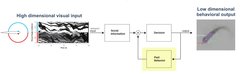
To relate sensory input to the movement decisions made by individuals we need to be able to identify appropriate motor output. In the two cases above behavioral transitions are relatively simple to classify, but in others there can be ambiguity regarding the behavioral state of individuals. We propose to resolve this problem by developing broadly applicable dimensionality-reduction techniques for identification of the low level structure of motor output employed when making movement decisions. This is related to ongoing work in other labs on mice and worms. A key challenge is to automatically identify low level motor elements, and how these are related to each other in sequences (analogous to deciphering the ‘language’ of behavioral elements). However developing such a representation of behavior is only part of the challenge – we also need to understand how the brain translates complex sensory input to these low level motor actions. We are investigating various machine learning methodologies to try to address this to facilitate a mapping between sensory input and motor output.