Quantitative approaches to the study of animal behaviour
A central thread that relates many of our studies is the development, and utilization of, new technologies for the observation and quantification of animal behavior. Collective animal behavior is inherently a multi-scale process, which is difficult, or impossible, to study using conventional observational techniques such as scan, and focal, sampling. Humans are highly subject to biases and inaccuracies when quantifying visual information. For over 15 years we have been developing, and utilizing, imaging technology for automated tracking of large number of animals in groups.
During my PhD (1999) I developed a realtime tracking system (in C++) for exploring, and foraging, ants which could infer possible interactions among individuals and measure kinematic properties.
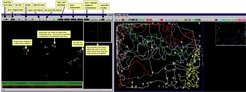
Following this, during my time as a postdoc, I developed a more general tracking tool, icBiovision, that has been used to quantify the movement of a large number of organisms including aphids, termites and locusts. A video of this in use for our locust work is shown here:
Since starting my lab we have been able to expand our technology through the amazing work of remarkable PhD students and postdocs. Particular thanks go to postdoc Hai Shan Wu, who now heads a group at Baidu, and PhD students Colin Twomey and Simon Leblanc. We are now able to track a very large number of deformable shapes, dealing with complex occlusion and identity issues. Below is a randomly selected individuals in a group of several hundred, showing this capability.
This allows us to obtain highly accurate motion data for large groups for arbitrarily long periods of time.
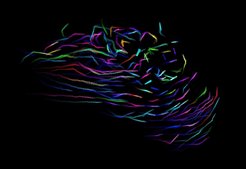
In addition to obtaining detailed positional information we also estimate the body posture of each individual, allowing us to undertake detailed analysis of the fine motor control exhibited by each individual.
This has allowed us infer which visual features are employed by individuals when making movement decisions, and to reconstruct the time-varying network of social interactions for free-swimming groups.
We would like to further enhance our capabilities, including 3D imaging (stereo video, lightfield imaging etc.) and analysis. If you are interested in this please get in touch!
Imaging has also been essential to our field studies. In our work on fish we have turned to acoustics to provide the raw data using high spatial and temporal resolution sonar (so-called ‘acoustic video’), as shown below.
which we have analyzed using computer vision (optical flow and related techniques) to establish the motion of both prey and predators.
For further information about this study see The dynamics of group hunting and collective evasion.
Another technology that we have adopted for field studies is global positioning system (GPS) tracking. For example we (Dr. Damien Farine, Ari Strandburg-Peshkin and I) recently worked with Prof. Meg Crofoot at UC Davis using high-resolution GPS to track the motion of baboons. Below is one of the baboons checking out a GoPro we were using to assess feeding behavior.
GPS tracking of the baboons allowed us to determine social influence in groups.
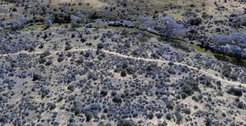
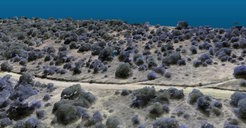
Dr. Mate Nagy in our group is also employing GPS to study the collective dynamics of pigeon flocks and we hope to have some of his work here soon.
Further quantitative approaches of great interest to our lab include machine learning (deep learning, dimensionality reduction etc.), trajectory analysis inference techniques for determining pathways of social influence in animal groups as well as computational approaches to infer the relationship between organisms and the complex habitat through which they move. It’s an exciting and dynamic time to be studying collective behaviour.